Today, NVIDIA released several production-ready, pre-trained models and a developer preview of Transfer Learning Toolkit (TLT) 3.0, along with DeepStream SDK 5.1. 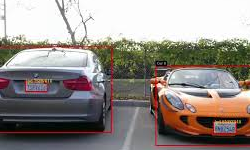
Intelligent vision and speech-enabled services have now become mainstream, impacting almost every aspect of our everyday life. AI-enabled video and audio analytics are enhancing applications from consumer products to enterprise services. Smart speakers at home. Smart kiosks or chatbots in retail stores. Interactive robots on factory floors. Intelligent patient monitoring systems at hospitals. And autonomous traffic solutions in smart cities. NVIDIA has been at the forefront of inventing technologies that power these services, helping developers create high-performance products with faster time-to-market.
Today, NVIDIA released several production-ready, pre-trained models and a developer preview of Transfer Learning Toolkit (TLT) 3.0, along with DeepStream SDK 5.1. The release includes a collection of new pre-trained models—innovative features that support conversational AI applications—delivering a more powerful solution for accelerating the developer’s journey from training to deployment.
Accelerate Your Vision AI Production
Creating a model from scratch can be daunting and expensive for developers, startups, and enterprises. NVIDIA TLT is the AI toolkit that abstracts away the AI/DL framework complexity and enables you to build production quality pre-trained models faster, with no coding required.
With TLT, you can bring your own data to fine-tune the model for a specific use case using one of NVIDIA’s multi-purpose, production-quality models for common AI tasks or use one of the 100+ permutations of neural network architectures like ResNet, VGG, FasterRCNN, RetinaNet, and YOLOv3/v4. All the models are readily available from NGC.
Key highlights for pre-trained models and TLT 3.0 (developer preview)
- New vision AI pre-trained models: license plate detection and recognition, heart rate monitoring, gesture recognition, gaze estimation, emotion recognition, face detection, and facial landmarks estimation
- Support for conversational AI use cases with pre-trained models for automatic speech recognition (ASR) and natural language processing (NLP)
- Choice of training with popular network architectures such as EfficientNet, YoloV4, and UNET
- Improved PeopleNet model to detect difficult scenarios such as people sitting down and rotated/warped objects
- TLT launcher for pulling compatible containers to initialize
- Support for NVIDIA Ampere GPUs with third-generation tensor cores for performance boost
Get Started
New Developer Webinar
Join the upcoming webinar “Using NVIDIA Pre-Trained Models and Transfer Learning Toolkit 3.0 to Create Gesture-based Interactions with a Robot” on March 3, 11 a.m. PT. We’ll demonstrate the entire end-to-end developer workflow in a video to show how easy the process is—from training to deployment—to build a gesture-recognition application with human-robot interaction. Register now >>
What Our Customers Are Saying
“INEX RoadView, our comprehensive automatic license plate recognition system for toll roads, uses NVIDIA’s end-to-end vision AI pipeline, production ready AI models, TLT, and DeepStream SDK. Our engineering team not only slashed the development time by 60% but they also reduced the camera hardware cost by 40% using Jetson Nano and Xavier NX. This enabled our vendors to deploy RoadView, the only out of the box ALPR solution, quickly and reliably. For us, nothing else came close.”
Dr. Roman Prilutsky, CEO/CTO, INEX
“We are enabling developers and third-party vendors to readily build intelligent AI apps leveraging Optra’s skills marketplace. As a new entrant to the Edge AI market, being able to differentiate our offerings and time to market was crucial. Readily available MaskRCNN from TLT and easy integration into DeepStream saved 25% development effort right out of the box for our R&D team.”
Chad McQuillen, Senior Technical Staff Member & Solutions Architect for Optra, Lexmark Ventures
“At Quantiphi, we use NVIDIA SDKs to build real-time video analytics workflows for many of our Fortune 500 customers across Retail and Media & Entertainment. Transfer Learning Toolkit provides an efficient way to customize training and model pruning for faster edge inference. DeepStream allows us to build high throughput inference pipelines on the Cloud and easily port them to the Jetson NX devices.”
Siddharth Kotwal, Solution Architecture Lead, Quantiphi
“KION Group is working on robust AI-based distribution autonomy solutions across its brands, to address operational needs and logistics optimization challenges and greatly reduce flow exception events. Innovation, engineering and digital transformation services are benefiting from optimized NVIDIA pre-trained models while rapidly innovating and fine-tuning models on the fly using Transfer Learning Toolkit and deploying with NVIDIA DeepStream unlocking multi-stream density with Jetson platforms.“
KION Group