![]() |
submitted by /u/iWatchBlack [visit reddit] [comments] |
New TensorBoard Integration in VS Code
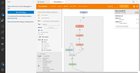
![]() |
In the latest update of VS Code last week, they added support for TensorBoard integration in VS Code. Just wanted to share with everyone! To launch tensorboard, just open the command palette in VS Code and search for the command “Launch TensorBoard” It looks like VS Code will automatically look for your TensorBoard log files within your directory. submitted by /u/evilcubed |
Help with debugging
![]() |
submitted by /u/iWatchBlack [visit reddit] [comments] |
New TensorBoard Integration in VS Code
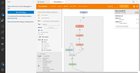
![]() |
In the latest update of VS Code last week, they added support for TensorBoard integration in VS Code. Just wanted to share with everyone! To launch tensorboard, just open the command palette in VS Code and search for the command “Launch TensorBoard” It looks like VS Code will automatically look for your TensorBoard log files within your directory. submitted by /u/evilcubed |
Help with debugging
![]() |
submitted by /u/iWatchBlack [visit reddit] [comments] |
New TensorBoard Integration in VS Code
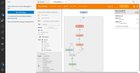
![]() |
In the latest update of VS Code last week, they added support for TensorBoard integration in VS Code. Just wanted to share with everyone! To launch tensorboard, just open the command palette in VS Code and search for the command “Launch TensorBoard” It looks like VS Code will automatically look for your TensorBoard log files within your directory. submitted by /u/evilcubed |
Help with debugging
![]() |
submitted by /u/iWatchBlack [visit reddit] [comments] |
New TensorBoard Integration in VS Code
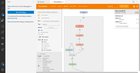
![]() |
In the latest update of VS Code last week, they added support for TensorBoard integration in VS Code. Just wanted to share with everyone! To launch tensorboard, just open the command palette in VS Code and search for the command “Launch TensorBoard” It looks like VS Code will automatically look for your TensorBoard log files within your directory. submitted by /u/evilcubed |
Help with debugging
![]() |
submitted by /u/iWatchBlack [visit reddit] [comments] |
NVIDIA today reported record revenue for the fourth quarter ended January 31, 2021, of $5.00 billion, up 61 percent from $3.11 billion a year earlier, and up 6 percent from $4.73 billion in the previous quarter. The company’s Gaming and Data Center platforms achieved record revenue for the quarter and year.